Greetings AI Thinkers,
It was a wild week in the world. According to Forbes, in an April 06, 2025 article, the stock market lost $9.6 trillion in value since January 17, 2025.
The world of AI has continued its progress with new developments, particularly significant updates from Google regarding agent-to-agent systems (see our writing about MCP), tools for building your applications (Firebase Studio), and more.
To stay updated, explore our AI leadership resources in our LinkedIn MindLi AI private Group
Feel free to share news there—we curate and maintain its quality.
Our “Spark of the Week” for leaders discusses the widely recognized fact— yet often overlooked — that AI can make mistakes. It will include several examples and tips for addressing this issue.
This also involves a cognitive shift in how we, as leaders, should perceive and explain AI-based digital systems.
Happy Thinking,
Dr. Yesha Sivan and the MindLi Team
P.S. Comments, ideas, feedback? Send me an e-mail.
Spark of the Week: Smart AI, Dumb Mistakes (Source: Yesha on Human Thinking)
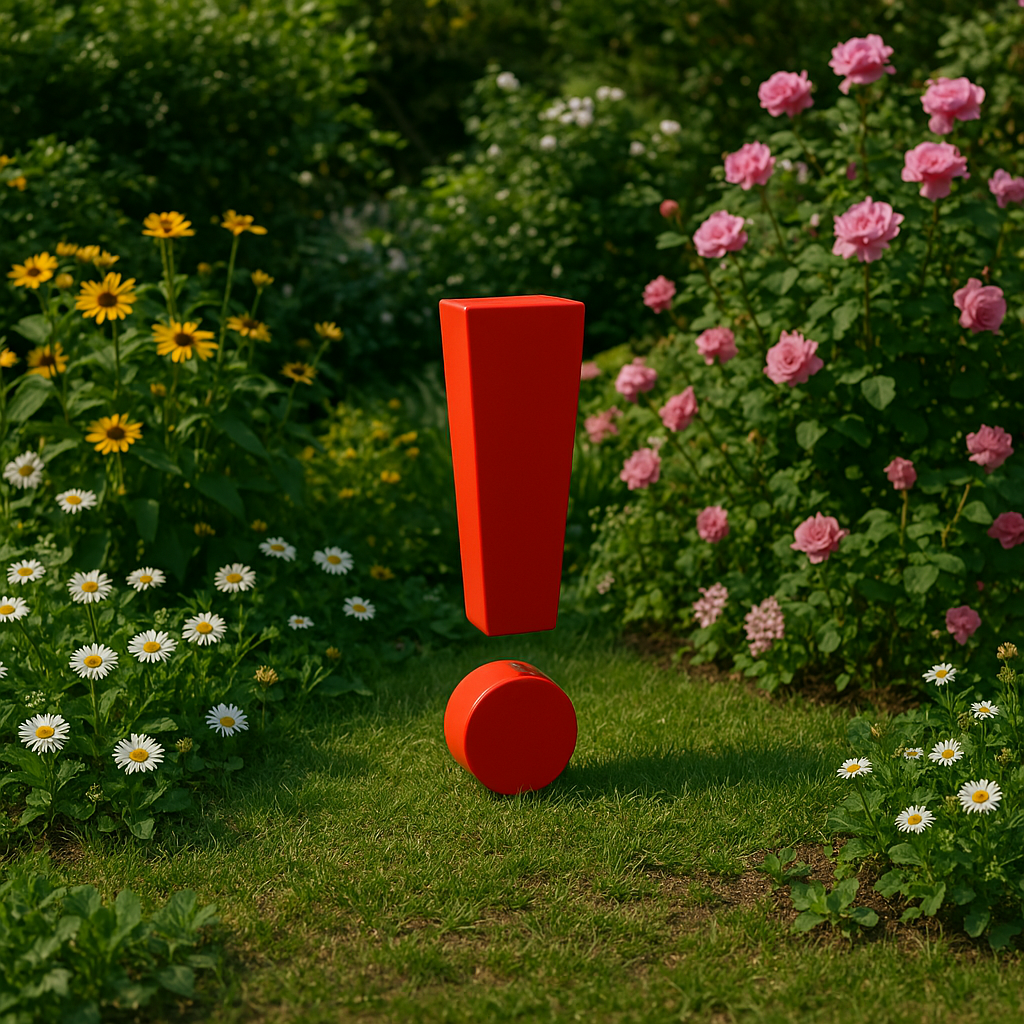
Opening: Factual, Logical, and Other Mistakes
Gaining the best value from AI as individuals and organizations requires first awareness and an understanding of the types of mistakes AI can make. Here are five telling examples.
Example 1: How Many R’s in a Strawberry
Until a few months ago, all significant LLMs would make a mistake when answering the question, “How many R’s are in strawberry? ” (See my post, “How many R letters are in the word strawberry?” Why do we need to check LLMs? Claude, GPT -4, and Gemini have an update on OpenAI: 1.0 – solved here.)
This morning, OpenAI GPT Model 4o gave me two options—2 or 3—and asked me what I preferred.
A simple question with a simple answer that the AI still can not answer correctly. And I ask: I’m paying for this?
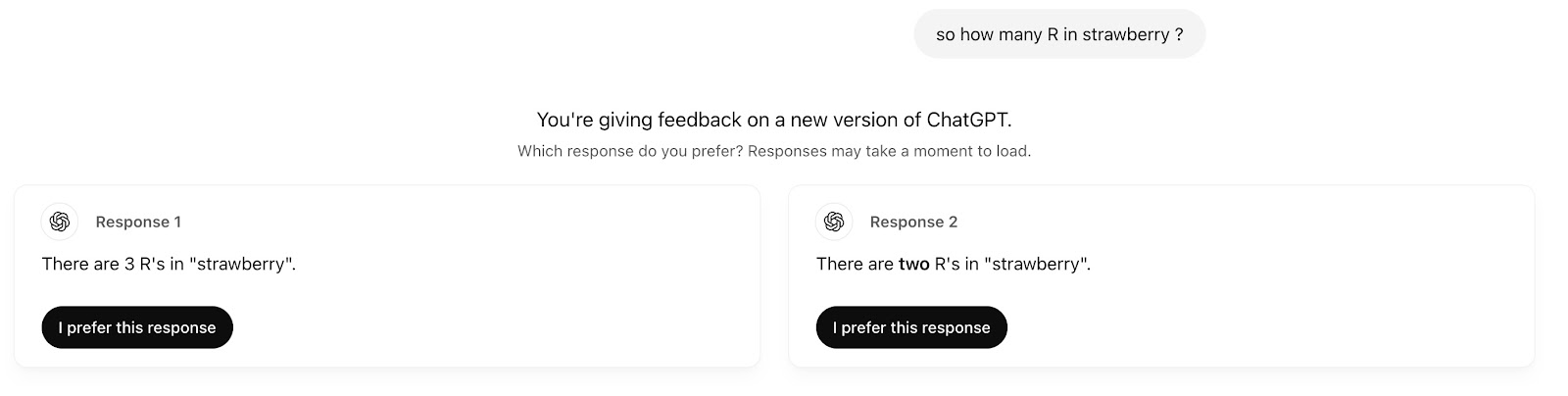
Example 2: Simple OCR and Simple Math
A helpful feature I have used for the last two years is asking GPT to help me summarize my monthly expenses. I gather the invoices, write down the sums, take a photo, and request a total. It usually works perfectly, and recently, it has become even faster.
That is why I was shocked when it failed to solve a simpler problem: taking the sum of numbers from an image of a spreadsheet (not handwriting).
Here is the image:
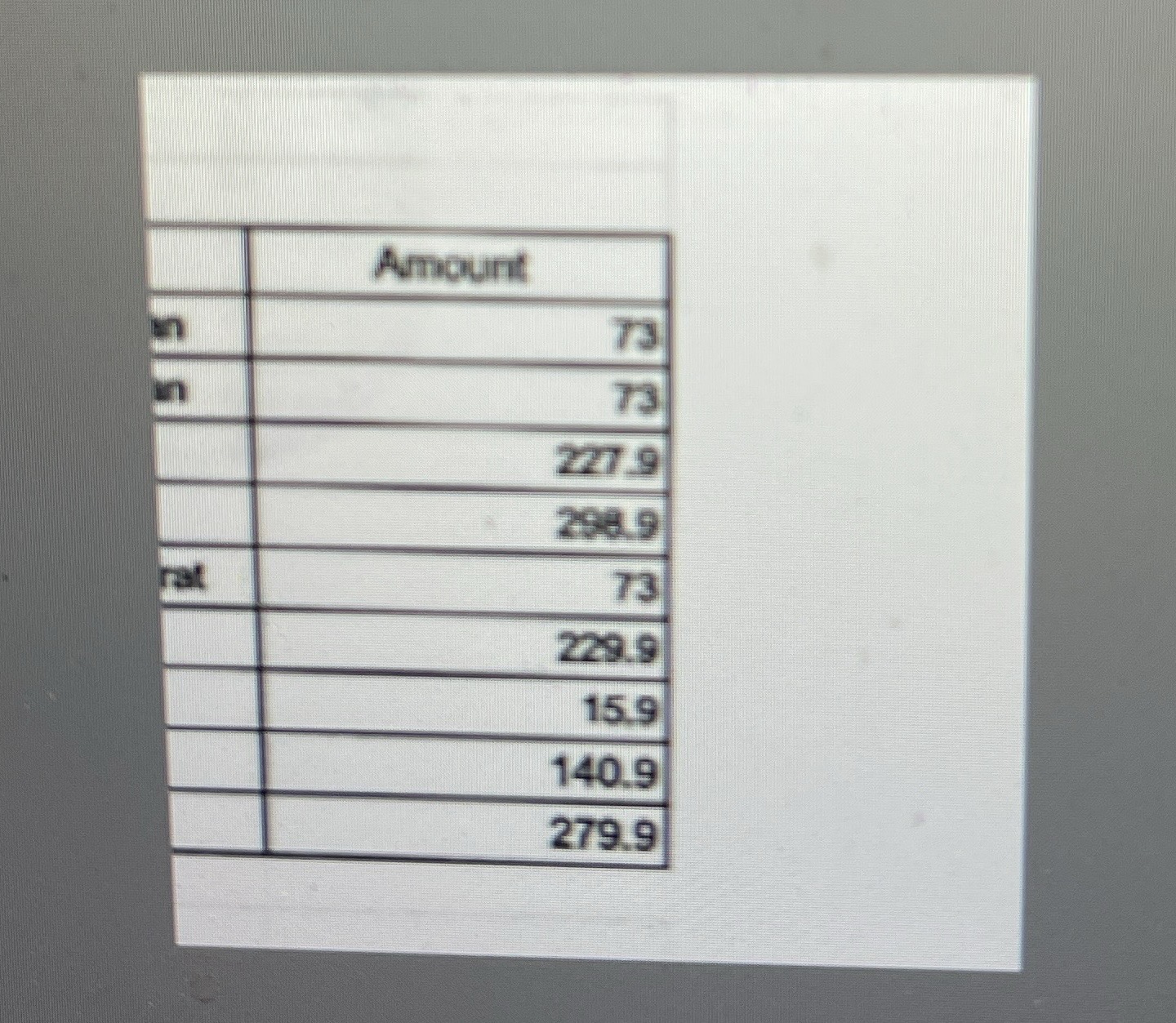
And here is the answer from GPT:
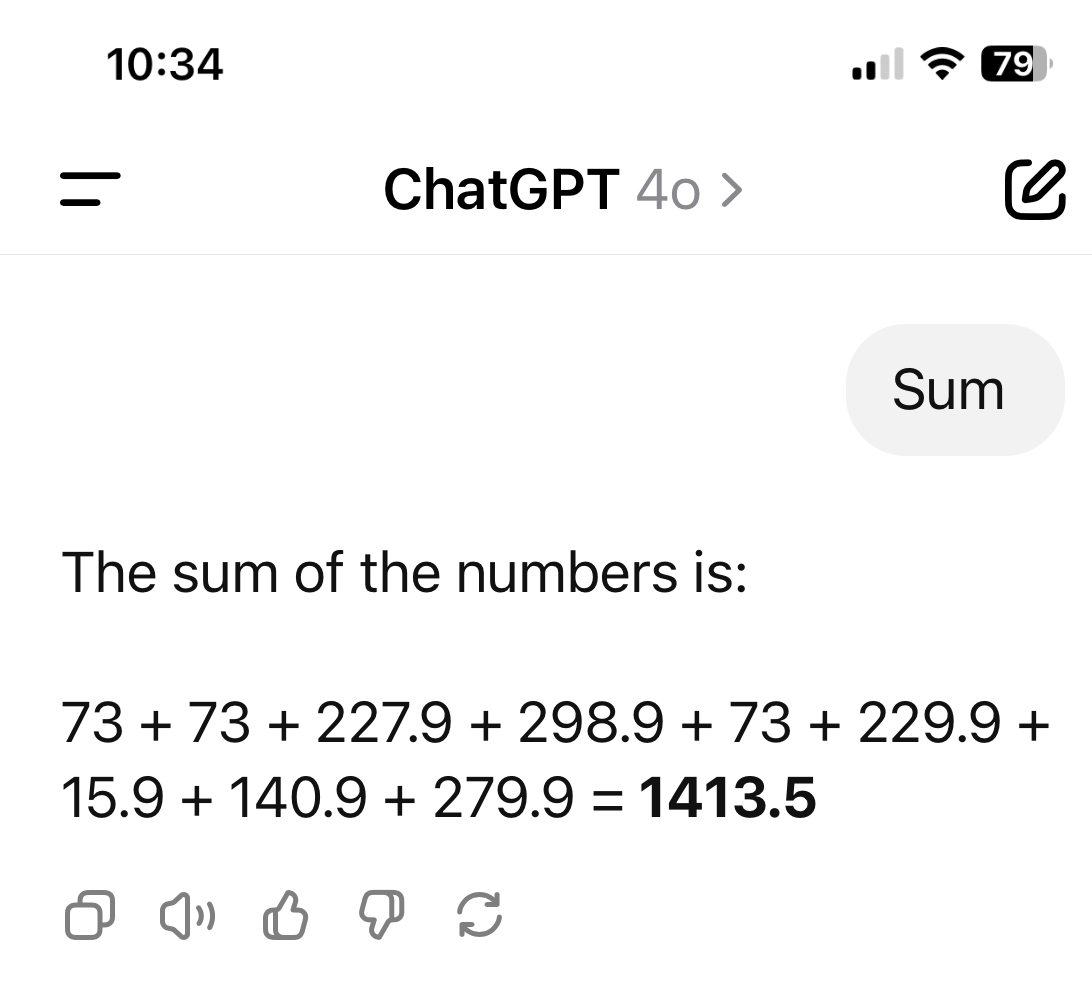
Impressive! Taking a scanned image and extracting all the numbers from within is cool. Also, understanding that’ sum” means to provide the total is great.
The only problem is that it provided the wrong answer. The correct answer is 1412.5, not 1413.5. It’s a simple addition error, as indicated by “asking GOT to check itself.” as seen here:
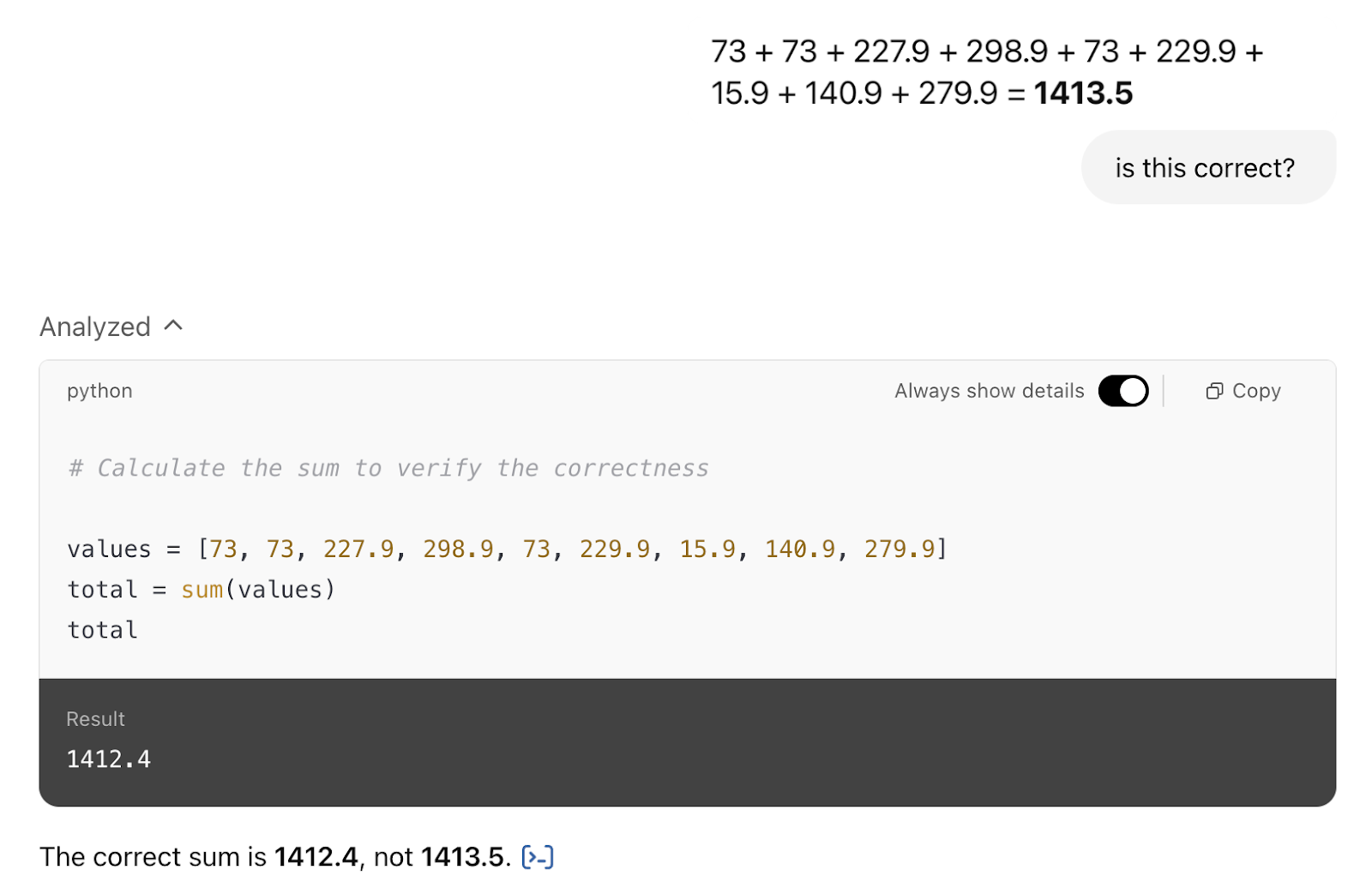
So, am I expected to check everything? My answer is yes.
Example 3: Letting AI Do Too Much: The Case of Pentagon Signal Leak
According to the Guardian (Hugo Lowell, Sun 6 Apr 2025 14.54 BST):
Donald Trump’s national security adviser, Mike Waltz, mistakenly included a journalist in the Signal group chat regarding plans for U.S. strikes in Yemen after he saved the wrong contact months earlier, according to three individuals briefed on the matter.
According to three individuals briefed on the internal investigation, Goldberg had emailed the campaign regarding a story that criticized Trump for his attitude toward wounded service members. To counter the story, the campaign enlisted the assistance of Waltz, their national security expert surrogate.
Goldberg’s email was forwarded to then-Trump spokesperson Brian Hughes, who then copied and pasted the content of the email – including the signature block with Goldberg’s phone number – into a text message that he sent to Waltz so that he could be briefed on the forthcoming story.
“… Waltz did not ultimately call Goldberg, the people said, but in an extraordinary twist, inadvertently ended up saving Goldberg’s number in his iPhone – under the contact card for Hughes, now the spokesperson for the National Security Council.”
In short, AI reads our emails, finds telephone numbers, and tries to help us connect with people. We accept this, and months later, the mistake explodes in our faces- because, in some cases, a phone number is a key.
Example 4: The Books I Should Write 🙂
The simplest way to show mistakes, at least in OpenAI, is to ask AI to give information you are deeply familiar with.
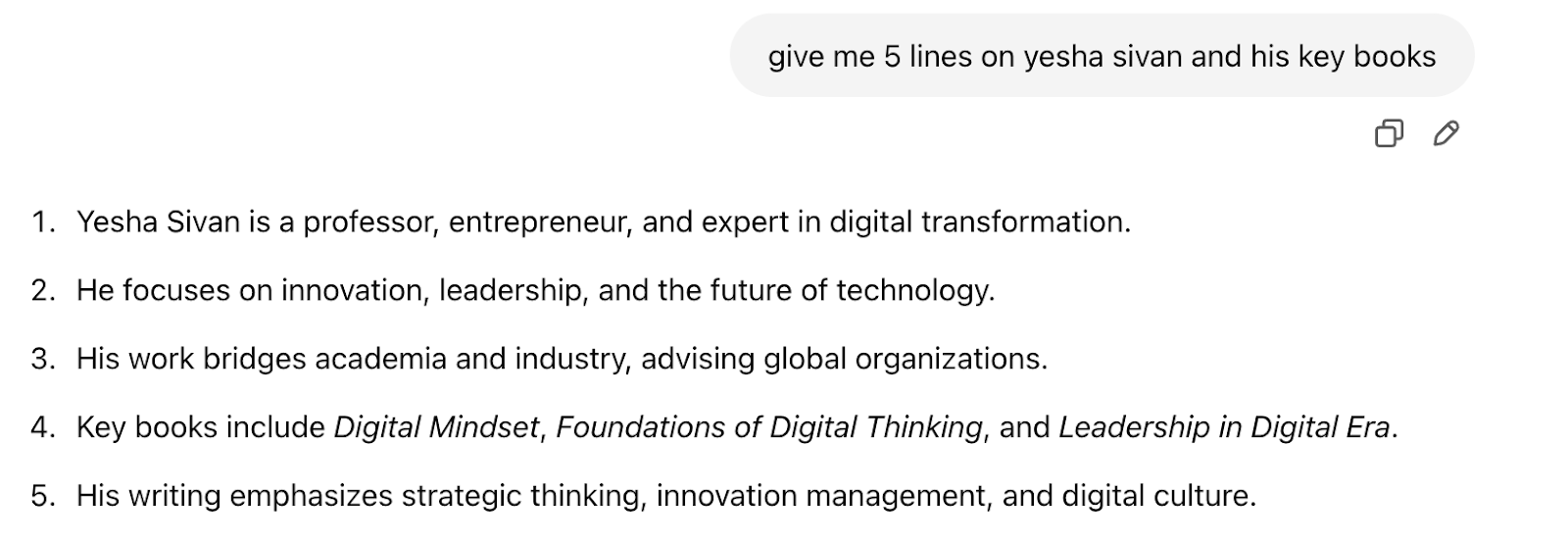
1, 2, 3, and 5 are acceptable; 4 is entirely incorrect.
I never wrote these books. By the way, if you ask for specific titles, ChatGPT apologizes: “Apologies for the earlier inaccuracies. Upon further research, here are the exact titles of key publications, and I will provide you with a correct answer.”
Example 5: A Trick That Saves Me Hours
For personal use, I collaborate with AI daily on various technical, programming, and development questions. In the early days, I took many wrong turns because the chat was sometimes inaccurate and misleading. The chat would create non-existent commands, reference outdated libraries, and even propose imaginary parameters.
That is why, for every technical solution, I always ask AI to “check again in another way” or, in this case, “check again the analysis of the expiry day.”
The exact details of the technical question are less critical here (it has to do with Apple tokens).
What is interesting is that AI analyzes a token (a set of letters) and extracts the coded date from it—albeit, as you can see from its own “check process again,” the original answer was simply mistaken.
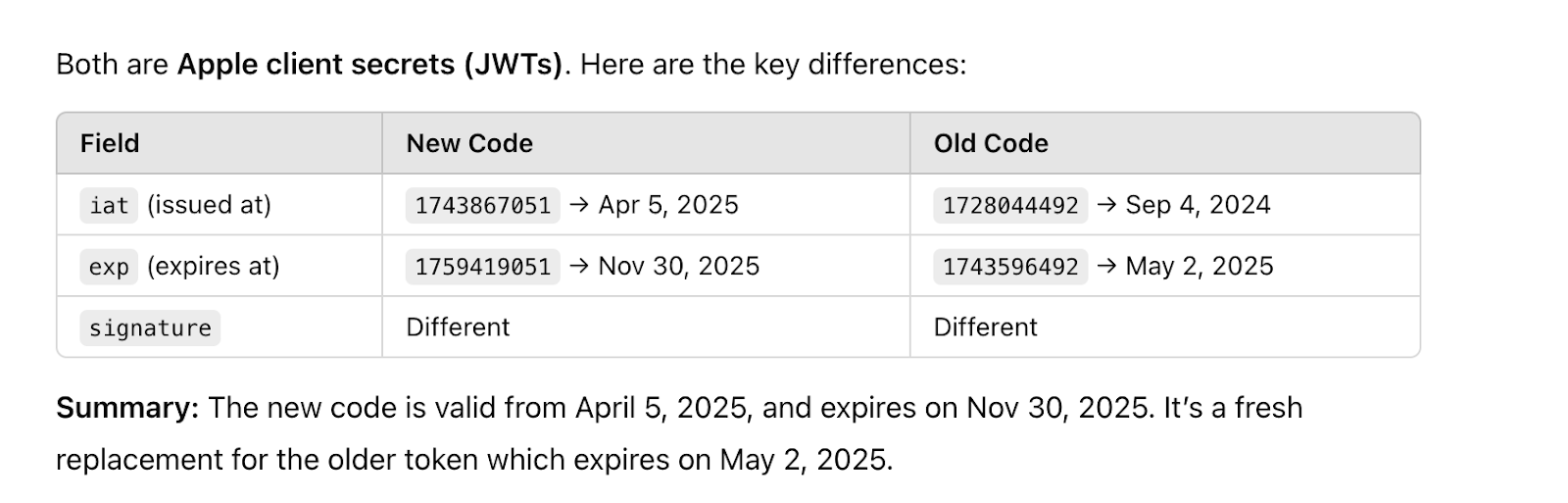
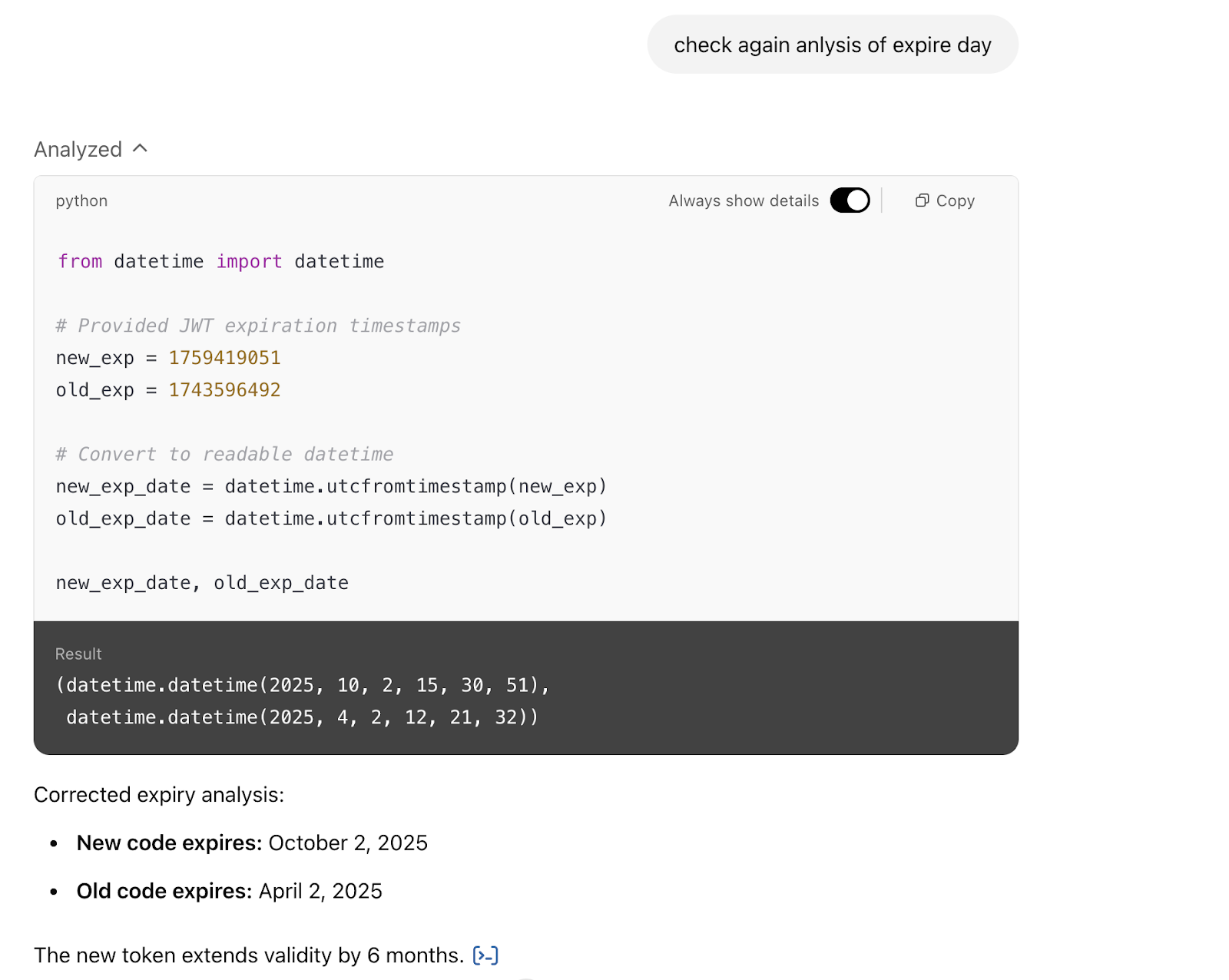
After confirming that the date had expired, a new token resolved the issue in 10 minutes. If I had trusted the chat’s initial response, I would have entered a debugging rabbit hole that could take hours. Be careful.
For Leaders: Key Shift From “The Algorithm That Knows” to “The Algorithm That Gives Us an Answer That We Should Check”
There are several reasons for such mistakes. Some stem from LLMs’ core workings (the way tokens are developed from words in the case of “the number of Rs in strawberry”), some from incorrect information fed to the LLM, and others from built-in randomness or the amount of time the LLM takes to consider questions.
Even worse, for complex issues, AI pundits often attribute blame to “emergent” phenomena. Emergence in LLMs constitutes a significant area of research that aims to clarify how LLMs function; hint: we are still uncertain.
What we know is that these systems can make mistakes. Moreover, we often receive different answers due to randomness, capacity, time of day, and A-B testing of the model. This necessitates a shift in mindset from “the algorithm knows” to “the algorithm provides an answer that we should verify.”
In a sense, we are returning to the foundational scientific method as defined by Karl Popper: the AI-enhanced world should progress by disproving incorrect ideas rather than by proving the correct ones.
P.S. In practical terms, always check your answers at least once. For critical things, check 2-3 more times using different LLMs.
About MindLi CONNECT Newsletter
Aimed at AI Thinkers, MindLi CONNECT newsletter is your news source and inspiration.
Enjoy!
MindLi – The Links You Need
General:
- Website — MindLi.com — All the details you want and need.
- LinkedIn — MindLi 🌍 GLOBAL Group — Once a week or so, main formal updates. ⬅️ Start here for regular updates.
- WhatsApp — MindLi Updates — If you need it, the same global updates will be sent to your phone for easier consumption. This is similar to the above Global group — once a week or so.
- Contact us – We’re here to answer questions, receive comments, ideas, and feedback.
Focused:
- LinkedIn — MindLi 🧠 AI Group — More technical updates on AI, AGI, and Human thinking. ⬅️ Your AI ANTI-FOMO remedy — Almost Daily.
- LinkedIn — MindLi 👩⚕️HEALTHCARE Group — Specifically for our favorite domain — healthcare, digital healthcare, and AI for healthcare — Weekly.
- LinkedIn — MindLi 🛠️ FOW – Future of Work Group — thinking about current and future work? This is the place for you — Weekly.
- LinkedIn — MindLi 🕶️ JVWR – Virtual Worlds Group — About virtual worlds, 3D3C, JVWR (Journal of Virtual World Research), and the good old Metaverse — Monthly.
- LinkedIn — MindLi Ⓜ️ Tribe Group — Our internal group for beta testers of MindLi, by invite — when we have updates, call for advice, need for testing, etc (also ask about our special WhatsApp group).